With the explosion of GenAI and foundation models, there is a growing debate about what the most important AI model will be in the months and years ahead. OpenAI’s GPT-4 is a clear market leader today, but its lack of openness, higher costs, and other customization restrictions make it an especially hard model for others to use at scale in production. Countless models with varying degrees of “openness” are vying to supplant GPT-4, including models from Meta, Amazon, Google, Microsoft, Anthropic, and Mistral. But, these debates are missing the key point. The most important model required to remain an enduring winner in AI is a compelling business model.
The proliferation of AI models has inspired a new generation of companies to leverage them and related technologies to solve customer problems. That customer focus is crucial, but it doesn’t work to just repackage other people’s technology if you’re not providing clear, differentiated value and building a sustainable moat! Many GenAI companies are experiencing rapidly changing fortunes and the need to evolve business models, as we’ve seen with Jasper, Stability AI, and Adept.
The world of applied AI is evolving so quickly that identifying the winning business model will require curiosity, iteration, and agility. Investors who have the company-building experience to recognize past patterns and thoughtfully apply them to this innovation cycle can help in that discovery journey. Below is a framework for thinking about business models, specifically the pricing and packaging approaches used by technology companies in recent eras. Based on previous disruptive technology cycles, including SaaS, mobile, and cloud native, it’s time to consider how these four approaches might apply to AI companies throughout the intelligent application stack.
Creating vs. Capturing Value
A compelling business model enables the creation and capture of value by offering solutions that address specific customer needs. It allows a startup to differentiate itself from competitors — and long-standing incumbents — by providing unique and differentiated offerings. Salesforce.com used this to its advantage with subscription pricing versus Siebel, which used a legacy license/maintenance pricing model. Business models not only facilitate profitable revenue generation but also support scalability and agility in an ever-changing technology sector, ultimately contributing to a company’s growth and success.
Successful business models are developed by exploring several key questions:
- Do you have a clear understanding of your target customer and the problem they are trying to solve
- Can you articulate the value created by your product in terms of the benefits it delivers to the customer
- Are you able to deliver a product/solution to your customer in both a cost-competitive and a differentiated way
- What pricing and packaging combination allows you to capture your fair share of the value created
Strategic pricing and packaging enable a startup to capture a portion of the value a customer receives. By thinking deeply about pricing and packaging, founders can test early whether their product/solution is beneficial and preferable in the customer’s eyes. Said more simply, pricing is a measure of strategic value. However, startups must align their cost of delivering value in a risk-adjusted manner – which is more complicated in the GenAI world due to the dependencies on scarce infrastructure capacity and the fixed commitments required by cloud providers. Startups are up against incumbents that already have data and customers — but we believe savvy founders will navigate the complexities of AI business models to become long-term winners.
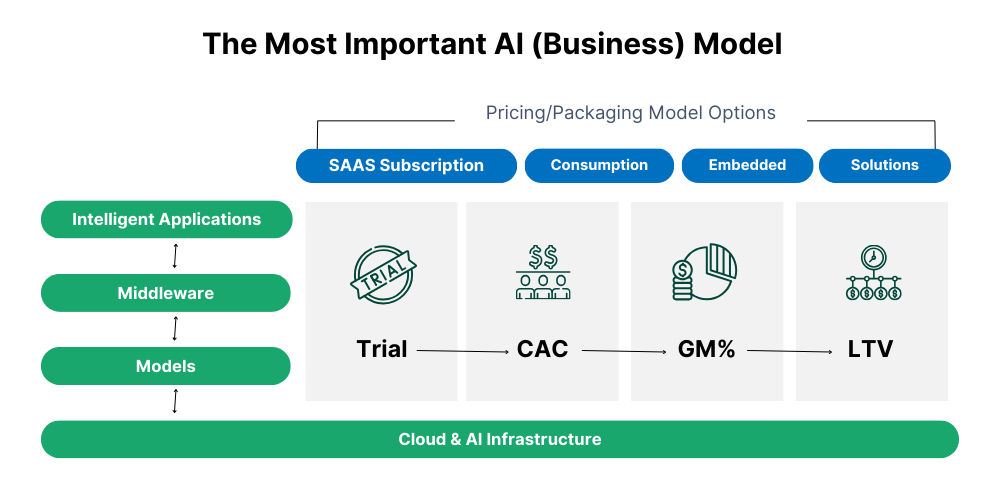
Business Models and the AI Stack
Subscription pricing
Subscription pricing appears to be the most compelling approach for startups building applications and copilot at the top of the AI Stack.
Subscription pricing models have dominated the software application world over the past two decades. These SaaS offerings are easily understood from a buyer and seller perspective. The customer often pays per user or “seat” — most sellers prefer offering a modest discount for an annual subscription commitment and upfront payment. Often, the customer gets to try the software through a free trial, a proof of concept, or a less-featured “forever free” version of the product. And, in an increasingly collaborative world, there are sometimes pricing distinctions between the seat prices of a user who creates content and those who contribute to a workflow or business process.
While subscription pricing is easy to understand and track, when it comes to intelligent applications, there are often higher underlying costs for the data, models, and compute required to train and run them. So, many software companies are experimenting with hybrid pricing models of a base subscription and then some consumption-based payment tied to model/token usage.
Consumption Pricing
Consumption models have grown in popularity for different technology services, including cloud infrastructure, data warehousing, and observability, and they are becoming more prevalent at the application layer. Consumption model pricing is tied to the underlying units of usage (e.g., tokens, compute, storage), and for GenAI, the underlying quality of AI models that power that software. These consumption units are generally tied to components of the solution that are variable in cost, so cost, value, and revenue are reasonably mapped. The alignment between the marginal cost of delivering a service (including their contracts with CSPs) and the price/value per unit charged for that service is often why technology providers prefer consumption pricing.
Consumption models have the disadvantage of not providing visibility into how much customers will be billed for the services they consume. There is often tension for customers between signing an annual, minimum-consumption contract to get discounts and visibility and enjoying the flexibility of a pure consumption model. An upfront commitment (often with cash upfront) mitigates some key flexibility benefits of the consumption model, but it guarantees access and preferred pricing.
Embedded Pricing
AI companies and their partners are exploring different forms of usage and distribution in the AI stack. Customers can access a model through an API or use a virtual private cloud (VPC) to access models and applications in a distributed manner. They can often access popular models through model aggregators like HuggingFace or AWS Bedrock for further customization and fine-tuning. Depending on where an AI company sits in the stack, it may choose to sell its product through another technology/distribution partner.
These approaches align with an “embedded” pricing model where the end user is not directly paying the model builder or enabling technology company for the value being delivered. In addition, some AI model aggregators run “models as a service” offerings and charge an end-user customer while paying a portion of those revenues to the model creator. Other AI model aggregators allow customers to select models and then run those models on the cloud service of their choice – in which case the cloud provider is sharing revenue back to the aggregator. There are other variations of these embedded models, and they are an alternative mechanism for players in the AI stack to access or monetize customers. We think the embedded approach will be one of the most innovative and dynamic areas of pricing, packaging, and distribution in the applied AI model era.
Intelligent application companies can also get embedded deals where an established software company has the primary relationship with the end user customer and embeds the AI-powered capabilities into their applications. Those capabilities can be embedded as an API into another app, such as the case with a transcription, voice-enabling, or translation service. In other cases, a full application, such as Microsoft’s Github or 365 Copilot, is combined with other software to enhance that existing app. The market for both application and model layer embedded opportunities is very early, and we expect it to evolve in the months ahead.
Solution Services and Pricing
Whether at the model, middleware, or application layer, AI services are often stand-alone and accessible to the end user customer (or the developer who is using them to build an app). As AI is applied to more specific functions or verticals, and new workflows require some “change management,” we see the rise of AI solution offerings. Model makers such as Anthropic and Cohere are working with clients to customize their domain-specific models in a secure (either on-premise or VPC) manner. Middleware companies are helping their customer select the best models, integrate them with other capabilities (such as a vector database for RAG), and then help with deployment. Gen-native application companies (Typeface and Runway in marketing, Harvey or Lexion in legal) often need to help their customer with onboarding, application personalization, and ongoing adoption/change management. We anticipate more elements of the whole solution being priced and packaged as service offerings, bundled with core subscription or consumption pricing, and sometimes combined with solution provider partner capabilities to help end user customers realize value.
Navigating the Road Ahead
As AI models proliferate, companies across the AI stack will need to think deeply about their business models in general and their pricing and packaging strategies in particular to ensure long-term success. There is currently a tension in AI business models between achieving near-term scale and delivering strong unit economics over time. This tension will become more acute as incumbents bundle AI with existing software and customers demand a clear ROI for the incremental dollars they are paying for AI to improve business processes or team productivity.
These are not new challenges. What is different is that very well-capitalized companies – the cloud providers – are working on two vectors of growth – building AI infrastructure AND backing emerging AI model players with billions of investments. The cloud providers are also trying to move “up market” to the middleware and application layer, as evidenced by Amazon Bedrock and Q, along with Microsoft copilots, GPT-4, and other domain-specific models. Model companies are creating new and improved models, and that proliferation is leading to value-capture uncertainty at the model layer. The role of Gen-native and Gen-enhanced applications appears to be favoring the incumbent software companies because they have the customers, the contextual data, and the workflows to build relevant AI improvements. However, a massive opportunity exists for a first-principled focus on customer needs, and we believe several AI-native companies will be able to navigate the complexities of business models to successfully provide customers with strategic value. These innovators will become long-term winners in the age of AI.